Data Analysis in Practice: Real-World Examples Explored
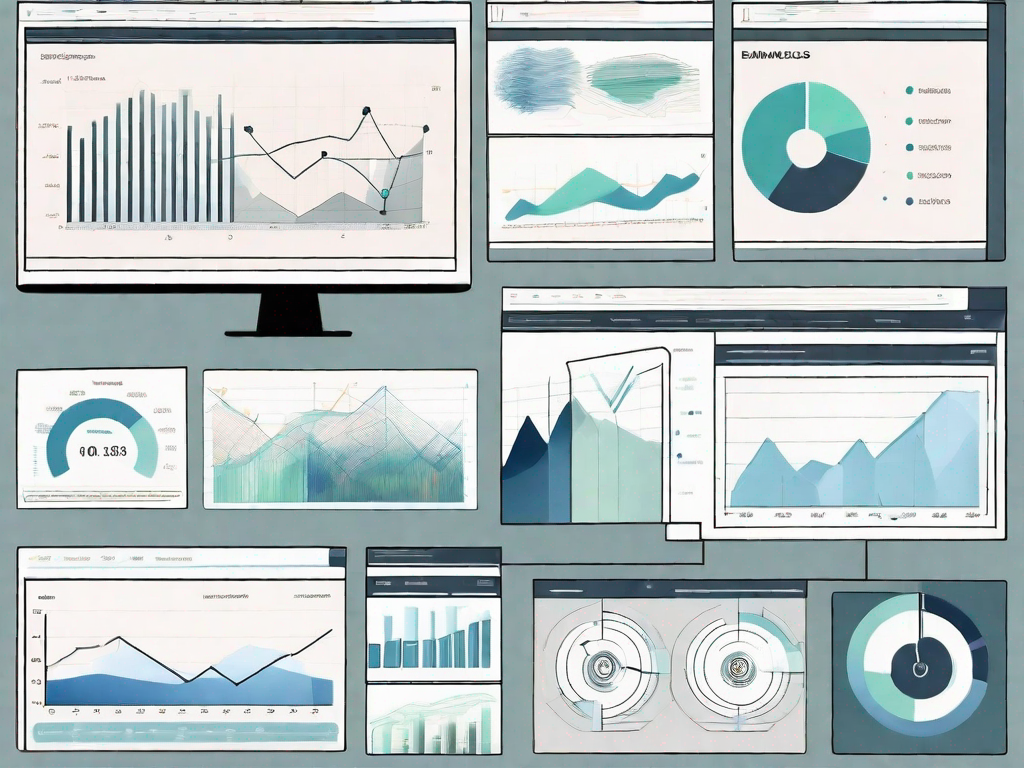
Data analysis is a critical process that helps businesses make informed decisions based on the insights gathered from data. In today's data-driven world, organizations of all sizes and industries rely on data analysis to drive growth, increase efficiency, and gain a competitive advantage. In this article, we will explore the basics of data analysis, the different types of analysis, the process involved, and real-world applications. Let's dive in!
Understanding the Basics of Data Analysis
Data analysis is a complex and systematic process that involves inspecting, cleansing, transforming, and modeling data to discover useful information, draw conclusions, and support decision-making. It goes beyond simply collecting data and involves various techniques and tools to extract meaningful insights from raw data.
Defining Data Analysis
At its core, data analysis is about making sense of the vast amount of information that is available to us. It allows us to uncover patterns, relationships, and trends that may not be immediately apparent. By carefully examining data, we can gain a deeper understanding of the world around us and make informed decisions based on evidence.
One of the key steps in data analysis is inspecting the data. This involves examining the data closely to identify any errors, inconsistencies, or missing values. By ensuring the quality of the data, we can have confidence in the results of our analysis.
Once the data has been inspected, it may need to be cleansed. This involves removing any irrelevant or duplicate data, as well as dealing with any outliers or anomalies. By cleaning the data, we can ensure that our analysis is based on accurate and reliable information.
After the data has been cleansed, it can be transformed. This involves manipulating the data in various ways to make it more suitable for analysis. For example, we may need to aggregate the data to a higher level or convert it into a different format. By transforming the data, we can uncover new insights and patterns that may not have been apparent in the original form.
Finally, the transformed data can be modeled. This involves applying statistical techniques or machine learning algorithms to the data to uncover relationships, make predictions, or classify data into different groups. By modeling the data, we can gain a deeper understanding of the underlying patterns and make more accurate predictions or decisions.
Importance of Data Analysis in Today's World
In today's world, data analysis plays a crucial role in various industries and sectors. It has become an essential tool for businesses, organizations, and governments to make informed decisions and stay competitive in a rapidly changing environment.
One of the key reasons why data analysis is important is its ability to help businesses understand customer behavior, preferences, and trends. By analyzing customer data, businesses can gain insights into what drives their customers' purchasing decisions, what products or services they prefer, and how they interact with the brand. This information can then be used to tailor products and services to meet customer needs, improve customer satisfaction, and ultimately drive business growth.
Furthermore, data analysis enables organizations to identify and solve problems. By analyzing data, organizations can detect anomalies or patterns that indicate potential issues or inefficiencies. This allows them to take proactive measures to address these problems, improve processes, and optimize performance. Data analysis also helps in making data-driven decisions, as it provides evidence and insights that support or challenge existing assumptions or beliefs.
In addition to problem-solving and decision-making, data analysis is also crucial for optimizing processes and improving operational efficiency. By analyzing data, organizations can identify bottlenecks, streamline workflows, and eliminate unnecessary steps or redundancies. This not only improves productivity but also reduces costs and improves overall performance.
Lastly, data analysis empowers businesses to stay ahead of competitors. By analyzing market data, businesses can uncover new market opportunities, identify emerging trends, and gain a deeper understanding of their industry. This allows them to develop innovative products or services, target new customer segments, and differentiate themselves from competitors.
In conclusion, data analysis is a powerful tool that enables us to make sense of the vast amount of information available to us. It involves a systematic process of inspecting, cleansing, transforming, and modeling data to uncover insights and support decision-making. In today's world, data analysis is essential for businesses and organizations to understand customer behavior, solve problems, optimize processes, and stay ahead of competitors.
Different Types of Data Analysis
Data analysis is a crucial process that helps businesses gain insights and make informed decisions based on the information they have. There are several types of data analysis techniques that serve different purposes. Let's explore some of the most common types:
Descriptive Analysis
Descriptive analysis aims to summarize and describe the main characteristics of a dataset. It involves calculating measures such as mean, median, mode, and standard deviation to understand the distribution and central tendencies of the data. Descriptive analysis provides a snapshot of the data and helps in generating insights about patterns, trends, and correlations.
For example, in a retail business, descriptive analysis can be used to understand the average purchase amount, the most popular products, or the demographics of the customers. By analyzing these descriptive statistics, businesses can identify key trends and make data-driven decisions to improve their operations.
Diagnostic Analysis
Diagnostic analysis focuses on understanding the causes and reasons behind specific outcomes or events. It involves identifying relationships between variables and determining the factors that contribute to certain outcomes. Diagnostic analysis helps businesses answer questions like "Why did this happen?" and provides a deeper understanding of the underlying factors driving specific outcomes.
For instance, in a healthcare setting, diagnostic analysis can be used to investigate the factors that contribute to patient readmissions. By analyzing patient data, such as demographics, medical history, and treatment plans, healthcare providers can identify patterns and risk factors that lead to readmissions. This information can then be used to develop strategies for reducing readmission rates and improving patient care.
Predictive Analysis
Predictive analysis utilizes historical data and statistical models to forecast future trends, behavior, or events. By analyzing patterns, relationships, and dependencies in data, predictive analysis helps businesses make informed predictions about future outcomes. This type of analysis is used in various fields, including sales forecasting, risk assessment, and demand prediction.
For example, in the financial industry, predictive analysis can be used to forecast stock prices based on historical market data. By analyzing past trends and patterns, financial analysts can build predictive models that estimate future stock prices. These predictions can then be used by investors to make informed decisions about buying or selling stocks.
Prescriptive Analysis
Prescriptive analysis goes beyond predictive analysis by recommending the best course of action based on data insights. It takes into account constraints, objectives, and available resources to provide decision-makers with actionable recommendations. Prescriptive analysis helps businesses optimize processes, allocate resources effectively, and make informed decisions in complex scenarios.
For instance, in supply chain management, prescriptive analysis can be used to optimize inventory levels and delivery routes. By considering factors such as customer demand, transportation costs, and warehouse capacities, businesses can use prescriptive analysis to determine the most efficient way to manage their supply chain. This can result in cost savings, improved customer satisfaction, and streamlined operations.
In conclusion, data analysis plays a vital role in helping businesses understand their data, gain insights, and make informed decisions. Whether it's descriptive, diagnostic, predictive, or prescriptive analysis, each type serves a specific purpose and contributes to the overall success of an organization.
The Process of Data Analysis
Data Collection
Data collection is the first step in the data analysis process. It involves gathering relevant data from various sources, such as surveys, databases, sensors, or social media platforms. Ensuring data quality and integrity is crucial during this stage to ensure accurate and reliable analysis.
Data Cleaning
Data cleaning, also known as data cleansing or data scrubbing, is the process of identifying and correcting or removing errors, inconsistencies, and inaccuracies in the collected data. This step ensures that the data used for analysis is accurate, complete, and consistent.
Data Interpretation
Data interpretation involves analyzing the cleaned data to extract meaningful insights and draw conclusions. It requires applying statistical techniques, visualization tools, and domain knowledge to understand patterns, relationships, and trends in the data.
Data Visualization
Data visualization is the process of representing data visually through charts, graphs, and other graphical representations. It enhances data understanding and communication by making complex information more accessible and easier to comprehend. Data visualization helps stakeholders grasp insights quickly and make data-driven decisions.
Real-World Applications of Data Analysis
Data Analysis in Healthcare
Data analysis has revolutionized the healthcare industry by enabling better patient care, disease prevention, and optimized resource allocation. With the help of data analysis, healthcare providers can identify high-risk patients, track the spread of diseases, predict outbreaks, and personalize treatment plans.
Data Analysis in Finance
In the finance industry, data analysis is used for risk management, fraud detection, investment decision-making, and customer segmentation. By analyzing financial data, organizations can identify market trends, optimize investment portfolios, detect fraudulent activities, and provide personalized financial advice to customers.
Data Analysis in Marketing
Data analysis is a powerful tool in the marketing world. It helps businesses understand customer behavior, preferences, and sentiment. By analyzing customer data, organizations can create targeted marketing campaigns, optimize pricing strategies, personalize customer experiences, and measure the effectiveness of marketing efforts.
In conclusion, data analysis is a fundamental process that brings meaning to data and helps organizations make informed decisions. With its various techniques, types, and real-world applications, data analysis has become an indispensable tool in today's competitive business landscape. By harnessing the power of data analysis, businesses can unlock valuable insights, optimize operations, and gain a strategic advantage in their respective industries.