How Long Does It Take to Become a Data Scientist?
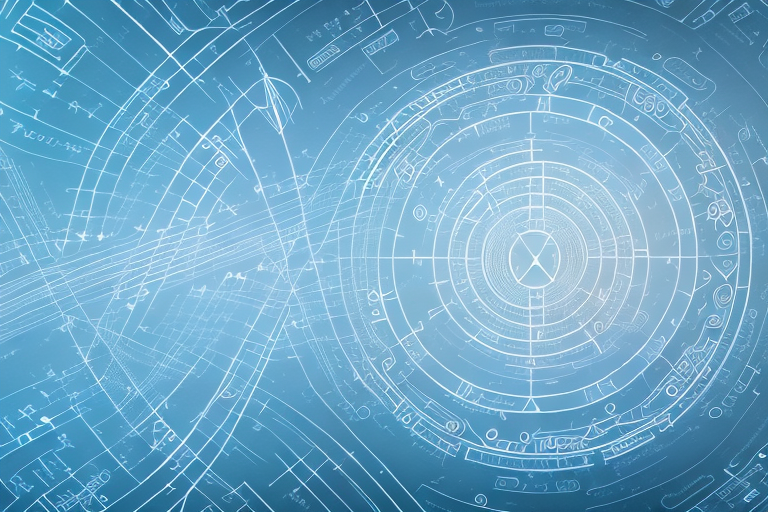
Becoming a data scientist can be a rewarding and lucrative career choice. However, it requires a significant investment of time and effort. If you're wondering how long it takes to become a data scientist, it's important to understand the role and responsibilities of a data scientist, the educational path to becoming one, the importance of practical experience, and the different specializations within the field.
Understanding the Role of a Data Scientist
Before diving into the timeline of becoming a data scientist, it's crucial to understand what the role entails. Data scientists are professionals who use their analytical and technical skills to extract insights and solve complex problems from vast amounts of data. They are responsible for handling, analyzing, and interpreting data to help businesses make data-driven decisions.
Data scientists play a pivotal role in today's data-driven world. They are the ones who uncover hidden patterns, trends, and correlations in data that can provide valuable insights for businesses. By leveraging their expertise in statistical analysis, programming, and domain knowledge, data scientists can help organizations optimize their operations, improve customer experiences, and drive innovation.
Key responsibilities of a data scientist include:
- Designing and implementing data-driven models and algorithms
- Developing predictive models for forecasting and trend analysis
- Exploring and visualizing data to identify patterns and trends
- Communicating findings and recommendations to stakeholders
Designing and implementing data-driven models and algorithms is a fundamental aspect of a data scientist's role. They use their expertise in machine learning, artificial intelligence, and statistical modeling to create models that can analyze and interpret complex datasets. These models help in making accurate predictions, identifying anomalies, and uncovering underlying patterns.
Another important responsibility of data scientists is developing predictive models for forecasting and trend analysis. By analyzing historical data and applying advanced statistical techniques, data scientists can forecast future trends and make informed predictions. These predictions can be used by businesses to optimize their strategies, allocate resources effectively, and stay ahead of the competition.
In order to identify patterns and trends in data, data scientists need to explore and visualize the data effectively. They use various data visualization techniques and tools to present complex data in a visually appealing and understandable manner. This helps stakeholders to grasp the insights easily and make informed decisions based on the data.
Communication is a vital skill for data scientists. They not only need to analyze and interpret data but also effectively communicate their findings and recommendations to stakeholders. This involves presenting complex technical concepts in a clear and concise manner, using visual aids, and tailoring the message to the audience. Effective communication ensures that the insights derived from data are understood and acted upon by decision-makers.
To excel in this role, data scientists need a combination of technical skills, domain knowledge, and a strong foundation in statistical analysis and programming. They should be proficient in programming languages such as Python or R, have a deep understanding of statistical concepts, and possess domain-specific knowledge. Additionally, data scientists should continuously update their skills and stay abreast of the latest advancements in data science to remain competitive in the field.
The Educational Path to Data Science
The educational path to becoming a data scientist typically involves obtaining a bachelor's degree in a relevant field such as mathematics, statistics, computer science, or engineering. Undergraduate studies provide a solid foundation in mathematics, programming, and statistical analysis, which are fundamental skills for a data scientist.
During their undergraduate studies, aspiring data scientists have the opportunity to delve into various subjects that form the building blocks of their future careers. They learn the intricacies of mathematical concepts like calculus, linear algebra, and probability theory, which are essential for understanding the statistical models and algorithms used in data science. Additionally, they gain proficiency in programming languages such as Python, R, and SQL, enabling them to manipulate and analyze large datasets efficiently.
Furthermore, undergraduate programs often offer specialized courses in data analysis and machine learning, where students learn how to apply statistical techniques and algorithms to extract meaningful insights from data. These courses provide hands-on experience with real-world datasets and equip students with the skills necessary to tackle complex data problems.
After completing the undergraduate degree, many aspiring data scientists choose to pursue postgraduate studies. A master's degree or a Ph.D. in a field like data science, analytics, or computer science can provide a more specialized and advanced education in areas such as machine learning, data mining, and data visualization.
Postgraduate programs offer a deeper exploration of advanced statistical methods, predictive modeling, and data visualization techniques. Students engage in research projects that allow them to apply their knowledge to real-world problems and contribute to the field's expanding body of knowledge. They also have the opportunity to collaborate with industry professionals and gain valuable insights into the practical applications of data science.
Moreover, postgraduate studies often involve internships or co-op programs, where students work alongside experienced data scientists in industry or research settings. These opportunities provide invaluable hands-on experience and allow students to apply their theoretical knowledge to real-world data challenges. They also enable students to build professional networks and establish connections that can be beneficial for future career prospects.
Overall, the educational path to data science is a comprehensive journey that combines theoretical knowledge with practical skills. It equips aspiring data scientists with the necessary tools to tackle complex data problems, make data-driven decisions, and contribute to the advancement of the field.
Importance of Practical Experience
In addition to formal education, practical experience is essential for becoming a successful data scientist. Internships and entry-level jobs in data analysis or related fields can provide valuable hands-on experience working with real-world data sets and solving practical problems.
One way to gain practical experience is through internships. These opportunities allow aspiring data scientists to work alongside professionals in the field, gaining insights into the day-to-day tasks and challenges they face. Interns may be involved in data collection, cleaning, and analysis, as well as assisting with the development of predictive models or machine learning algorithms.
Furthermore, entry-level jobs in data analysis provide a platform for individuals to apply their theoretical knowledge to real-world scenarios. These positions often involve working with large datasets, performing statistical analysis, and generating reports or visualizations to communicate findings to stakeholders. By working on actual projects, data analysts can develop a deeper understanding of data manipulation techniques, programming languages, and analytical tools.
Another crucial aspect of gaining practical experience is building a portfolio. Data scientists should showcase their projects, research, and analysis to demonstrate their capabilities and expertise. This can include creating interactive visualizations, publishing articles or blog posts about data-driven insights, or contributing to open-source projects.
Creating interactive visualizations allows data scientists to present complex information in a user-friendly and engaging manner. By utilizing tools such as Tableau or D3.js, they can develop interactive dashboards or charts that allow users to explore the data and draw their own conclusions. These visualizations not only demonstrate technical skills but also showcase the ability to communicate data-driven insights effectively.
In addition to visualizations, publishing articles or blog posts about data-driven insights can help data scientists establish themselves as thought leaders in the field. By sharing their research findings, methodologies, and insights, they contribute to the broader data science community and demonstrate their expertise in specific domains or industries.
Contributing to open-source projects is another way for data scientists to gain practical experience and showcase their skills. Open-source projects provide opportunities to collaborate with other data scientists, work on real-world problems, and contribute to the development of cutting-edge tools and algorithms. By actively participating in these projects, data scientists can demonstrate their ability to work in a team, adapt to different coding styles, and contribute to the advancement of the field.
In conclusion, practical experience is crucial for aspiring data scientists. Internships and entry-level jobs provide hands-on experience and the opportunity to apply theoretical knowledge to real-world scenarios. Building a portfolio through interactive visualizations, publishing articles or blog posts, and contributing to open-source projects helps data scientists showcase their capabilities and establish themselves as experts in the field.
Specializations in Data Science
Data science is a broad field with various specializations. While all data scientists need a solid foundation in statistics and programming, specializing in specific areas can help professionals stand out and find their niche within the industry.
Some common specializations in data science include:
- Machine Learning Specialist: Focusing on developing and implementing machine learning algorithms to analyze and predict patterns in data.
- Data Engineering Specialist: Specializing in designing and implementing data infrastructure and pipelines to collect, process, and store data efficiently.
The Timeline to Becoming a Data Scientist
The timeline to becoming a data scientist can vary depending on individual circumstances and career goals. The following factors should be considered:
Time Required for Education
Obtaining a bachelor's degree typically takes four years, while a master's or Ph.D. can add an additional two to six years of study. However, it's important to note that formal education alone may not be sufficient. Continuous learning and staying up-to-date with the latest tools and techniques are essential in the fast-evolving world of data science.
Time Required for Gaining Experience
Building practical experience through internships or entry-level jobs can take anywhere from several months to a year or more. Developing a portfolio with significant projects and contributions may also require additional time and effort.
In conclusion, becoming a data scientist requires a significant investment of time and effort. While the timeline can vary, it typically involves completing a bachelor's degree, pursuing postgraduate studies, gaining practical experience through internships or entry-level jobs, and specializing in specific areas of data science. Continuous learning and staying up-to-date with the latest advancements are also crucial for success in this dynamic field.