The Integral Role of Statistics in Data Science for Beginners
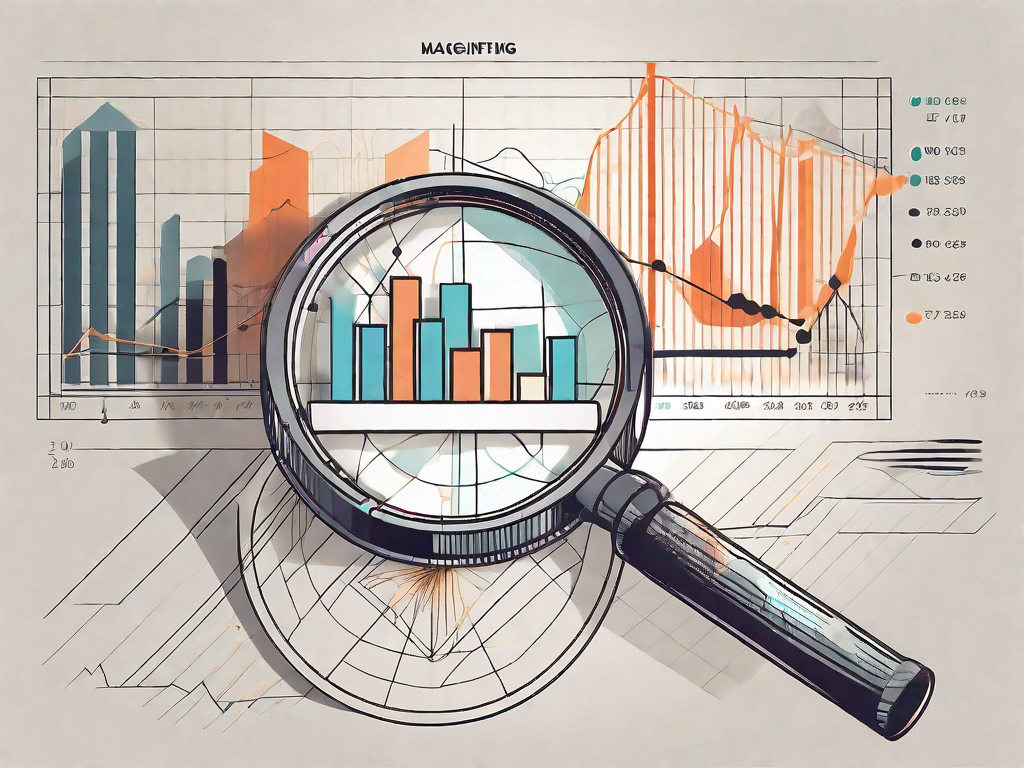
Data Science has emerged as a transformative field in the digital age, revolutionizing the way we approach decision-making and problem-solving. At the foundation of this discipline lies Statistics, playing a vital role in uncovering insights, making accurate predictions, and driving data-driven decisions. Whether you are new to Data Science or looking to strengthen your understanding, gaining proficiency in Statistics is essential. In this article, we will explore the basics of Data Science, delve into fundamental statistical concepts, and highlight the significance of statistical thinking in this rapidly evolving field.
Understanding the Basics of Data Science
Data Science is a multidisciplinary field that combines elements of mathematics, statistics, computer science, and domain expertise to extract actionable insights from data. It involves the collection, cleaning, analysis, and interpretation of complex datasets to uncover patterns, trends, and relationships that can drive informed decision-making. At the heart of Data Science lies statistics, providing the framework for data analysis and interpretation.
Data Science has gained significant prominence in recent years due to the exponential growth of data and the increasing need for organizations to make data-driven decisions. With the advent of technology and the proliferation of digital platforms, massive amounts of data are being generated every second. This data holds valuable information that can be harnessed to gain a competitive edge in various industries.
One of the key aspects of Data Science is the ability to collect and clean data effectively. This process involves sourcing data from various sources, such as databases, APIs, or even scraping data from websites. Once the data is collected, it needs to be cleaned and preprocessed to remove any inconsistencies, errors, or missing values. Data cleaning is a crucial step as it ensures that the subsequent analysis is based on accurate and reliable data.
Defining Data Science and Its Importance
Data Science refers to the process of extracting knowledge and insights from data through scientific methodologies, algorithms, and systems. It enables organizations to gain a competitive edge by leveraging data to optimize processes, improve products and services, and enhance customer experiences. By employing statistical techniques, Data Scientists can explore vast amounts of data, identify meaningful patterns, and make accurate predictions.
One of the key reasons why Data Science is important is its ability to uncover hidden patterns and trends in data. These insights can help businesses identify new opportunities, understand customer behavior, and make data-driven decisions. For example, in the e-commerce industry, Data Science can be used to analyze customer purchase history and preferences to personalize product recommendations, leading to increased sales and customer satisfaction.
Moreover, Data Science plays a crucial role in the field of healthcare. By analyzing large healthcare datasets, Data Scientists can identify risk factors, predict disease outbreaks, and develop personalized treatment plans. This can significantly improve patient outcomes and contribute to the overall advancement of medical research.
The Intersection of Data Science and Statistics
Data Science and Statistics are interconnected disciplines, each bolstering the other's capabilities. While Data Science encompasses a broader set of tools and techniques, statistics provides the foundation for hypothesis testing, sampling, probability, and regression analysis. Understanding statistical concepts is crucial for Data Scientists to turn raw data into insights and actionable recommendations.
Statistics provides Data Scientists with the necessary tools to make inferences and draw conclusions from data. By applying statistical techniques, Data Scientists can determine the significance of their findings, assess the reliability of their models, and quantify the uncertainty associated with their predictions. These statistical methods help ensure that the insights derived from data are robust and reliable.
Furthermore, statistics enables Data Scientists to make accurate predictions and forecasts. By analyzing historical data and identifying patterns, Data Scientists can develop predictive models that can forecast future trends and outcomes. These predictions can be invaluable for businesses in making strategic decisions, optimizing resources, and mitigating risks.
In conclusion, Data Science and Statistics are intertwined fields that complement each other in extracting insights from data. While Data Science provides the tools and techniques to analyze and interpret complex datasets, statistics provides the foundation for hypothesis testing, probability, and making accurate predictions. Together, these disciplines empower organizations to harness the power of data and make informed decisions that drive success.
The Fundamental Concepts of Statistics
Statistics is the branch of mathematics that deals with the collection, analysis, interpretation, presentation, and organization of data. It involves various techniques that enable us to summarize, describe, and draw meaningful inferences from data. Let's explore two essential concepts in statistics - Descriptive Statistics and Inferential Statistics.
Descriptive Statistics: A First Step in Data Analysis
Descriptive Statistics involves the analysis and summary of data to provide a clear and concise understanding of its main characteristics. Through measures like mean, median, mode, and standard deviation, Descriptive Statistics allows us to describe and visualize data, identifying central tendencies, variability, and distributions. By organizing data using charts, graphs, and tables, Data Scientists can quickly grasp its inherent patterns and outliers.
For example, consider a dataset that contains information about the heights of individuals in a population. Descriptive Statistics can help us determine the average height, the most common height, and the range of heights within the population. By calculating the mean height, we can get an idea of the central tendency, while the standard deviation can provide insights into the variability of heights. Visualizing this data using a histogram or a box plot can further enhance our understanding of the distribution of heights.
Furthermore, Descriptive Statistics can also be used to identify outliers in the data. Outliers are data points that significantly deviate from the rest of the dataset. These outliers may indicate measurement errors, data entry mistakes, or even rare occurrences. By detecting and analyzing outliers, Data Scientists can gain valuable insights into potential data quality issues or unique phenomena within the dataset.
Inferential Statistics: Making Predictions from Data
Inferential Statistics goes beyond descriptive analysis, enabling us to make inferences and predictions about a larger population based on a sample. Through techniques like hypothesis testing and confidence intervals, Data Scientists can make statistically significant conclusions about relationships, differences, and causal effects within the data. Inferential Statistics is crucial for generalizing findings from a sample to make predictions or draw conclusions applicable to a broader population.
For instance, imagine a scenario where a pharmaceutical company wants to test the effectiveness of a new drug. They cannot administer the drug to the entire population, so they select a sample of individuals to participate in the study. By applying Inferential Statistics, the company can analyze the data from the sample and draw conclusions about the effectiveness of the drug for the entire population. Hypothesis testing can help determine if there is a significant difference in outcomes between the group that received the drug and the group that received a placebo.
Inferential Statistics also allows Data Scientists to estimate population parameters with a certain level of confidence. Confidence intervals provide a range of values within which the true population parameter is likely to fall. This helps quantify the uncertainty associated with the estimates and provides a measure of reliability for the conclusions drawn from the sample data.
Moreover, Inferential Statistics plays a crucial role in various fields, such as market research, social sciences, and public health. It enables researchers to make informed decisions, develop effective strategies, and draw meaningful insights from limited data.
The Role of Probability in Statistics and Data Science
Probability theory plays a vital role in both Statistics and Data Science, providing the mathematical foundation for uncertainty and randomness. By quantifying the likelihood of events, Probability enables Data Scientists to make informed decisions, model uncertainties, and measure the reliability of conclusions drawn from data.
Probability Theory Basics
Probability theory is concerned with quantifying uncertainty and determining the likelihood of events occurring. It involves concepts such as sample spaces, events, probability distributions, and random variables. By understanding these fundamental principles, Data Scientists can effectively model real-world scenarios and make probabilistic predictions.
How Probability Drives Statistical Analysis
Probability is the backbone of Statistical Analysis as it provides the tools to estimate parameters, test hypotheses, and make predictions. Through techniques like Bayesian Inference, Data Scientists can update their beliefs and quantify uncertainty as new data becomes available. Probability empowers statistical models, enabling us to understand the inherent uncertainty in any analysis and make reliable judgments based on data.
Statistical Techniques in Data Science
Data Science relies heavily on various statistical techniques to uncover insights and solve complex problems. Let's explore two commonly used techniques - Regression Analysis and Hypothesis Testing.
Regression Analysis and Its Applications
Regression Analysis is a statistical technique used to model and analyze the relationship between a dependent variable and one or more independent variables. It enables Data Scientists to understand and predict how changes in independent variables affect the outcome of interest. Regression Analysis finds applications in diverse fields like economics, finance, marketing, and social sciences.
Hypothesis Testing in Data Science
Hypothesis Testing is a statistical method used to make inferences about a population based on sample data. It involves formulating a null hypothesis and an alternative hypothesis, collecting sample data, and determining the likelihood of observing the given data under the null hypothesis. Hypothesis Testing helps Data Scientists make objective decisions, evaluate the significance of relationships, and validate assumptions.
The Importance of Statistical Thinking in Data Science
Beyond the technical knowledge of statistical techniques, developing a statistical mindset is critical for successful Data Science. Statistical thinking involves approaching problems with a skeptical and analytical perspective, considering uncertainty, questioning assumptions, and embracing a data-driven approach.
Developing a Statistical Mindset
A statistical mindset encourages Data Scientists to be critical thinkers and problem-solvers. By focusing on data quality, properly framing questions, and accounting for confounding variables, they can ensure accurate and reliable analysis. Employing statistical thinking allows for robust decision-making, minimizing biases and maximizing the impact of data-driven insights.
The Impact of Statistical Thinking on Data-Driven Decisions
Statistical thinking empowers organizations to make informed decisions based on data. By embracing uncertainty and understanding the limitations of analysis, Data Scientists can communicate the risks and trade-offs involved in different options. Statistical thinking enables businesses to optimize processes, allocate resources effectively, and strategize for the future, leading to improved performance and competitive advantage.
Aspiring Data Scientists and beginners in the field must recognize the integral role of Statistics in Data Science. By mastering fundamental statistical concepts, understanding the intersection of Data Science and Statistics, and developing a statistical mindset, they can navigate the vast landscape of data and extract valuable insights. Statistics remains a cornerstone of Data Science, providing the tools and techniques necessary to transform raw data into actionable knowledge.